One of the big issues with large language models that sit behind generative AI applications is that we don’t know much about their dietary habits. What data were they fed — public or private? Was the data acquired legally or perhaps illicitly? Is the language model functionality optional in the app, or there’s no way of turning it off?
Case in point—for several months my team was unable to use OpenAI’s API to GPT-3, because the creators wouldn’t reveal what happens to the data we would have to submit to the API when running our own generative AI applications based on the model. This was a major showstopper, as our research integrity rules limit us regarding what we can do with the data we collect in our projects.
Echoes from Other Industries
These questions are not dissimilar to what customers of other industries ask. What is the energy efficiency of a car I want to buy? How much sugar is in this “sports” drink?
In light of these challenges, the 90s witnessed the birth of nutrition labelling, initially in the U.S., and then most countries followed suit. Similarly, car manufacturers globally now must display labels showing energy consumption and CO₂ emissions.
The beauty of these labels isn’t just the insight they offer about the product; they also steer our focus towards broader issues — be it moderating sugar intake or curbing CO₂ production.
Peeling Back the AI Label
As you’ve read in my earlier newsletter (Going MAD), we need to tread carefully with Generative AI apps, partly because the lines between traditional and AI-powered applications have become blurred (is Google search powered by Generative AI, or not?), and we’re often not sure what happens behind the user interface.
And so I was very excited to see the emergence of the first “nutrition” labels for Generative AI applications.
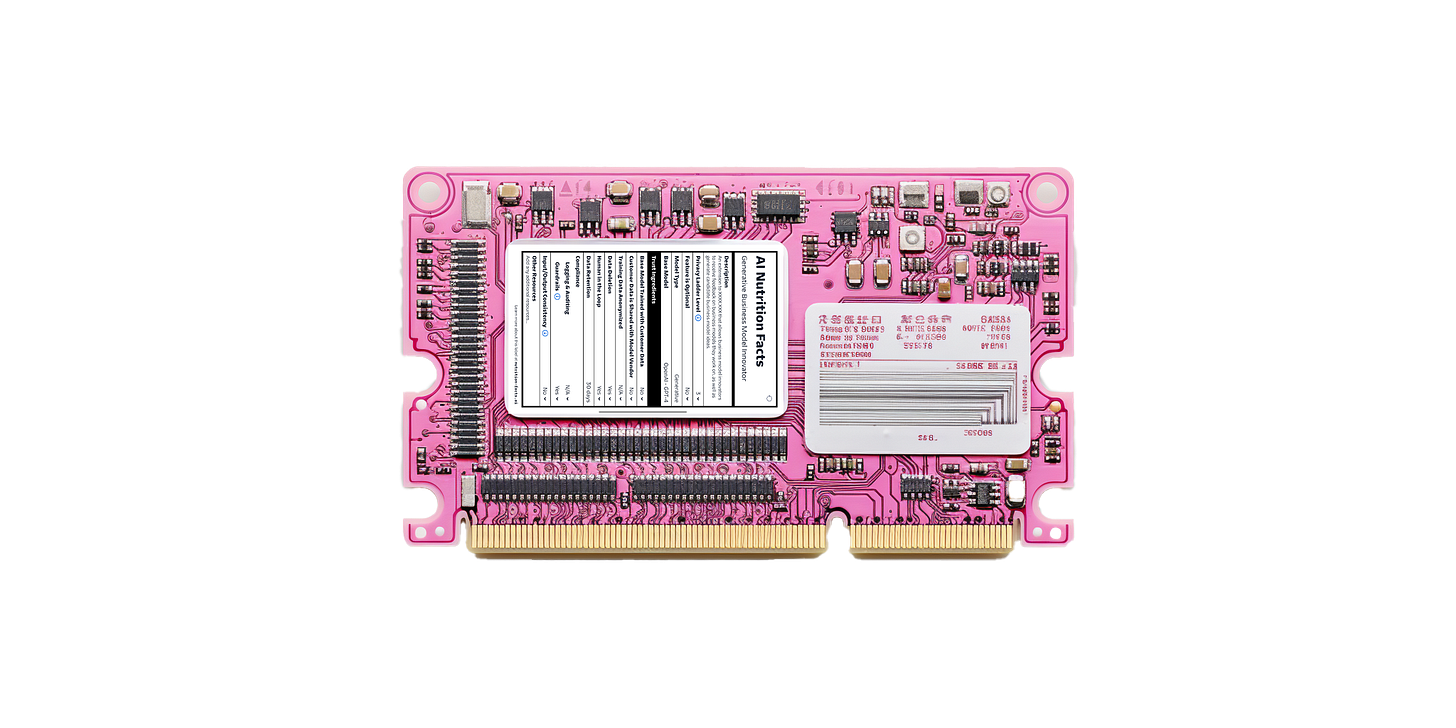
Nutrition-facts dot ai
Two weeks ago, Twilio launched a new website, nutrition-facts.ai. This platform lets software developers create standardised descriptions for their generative AI powered applications, similar in style to nutrition labels.
It’s a simple-to-use template that anyone can quickly fill out to provide essential information about their app.
What’s on the Menu?
Privacy: developers can indicate whether their models include personally identifiable information, and whether these models are accessible only to you (or your organisation) or to others too. For instance, some data we collect and use in our research should never be accessible outside of the institution. An appropriate privacy level (Level 1 or Level 2 in the case of Twilio labels) would need to be maintained.
Optionality: some applications might be using Generative AI as a feature, and it is possible to simply not use AI-powered features, but still use the app. For instance, I use Adobe’s Photoshop with Generative AI extensions, but when I need to ensure that my creations do not contain any artifacts created by Generative AI, I can use Photoshop’s “standard” functionality, ignoring the Generative AI tools.
Model type and its specific instance: not every AI application is a generative AI application. The label can clarify what it is, and what model specifically is being used. For example, if you’re using an image generation application powered by Midjourney, you might prefer an app using Midjourney version 5.2, over Midjourney version 2.
Trust ingredients: this section of the label dives deeper into what happens with the inputs and outputs. Will the model train on customer-provided data or not? Many organisations will prefer a read-only access, where they send their data to generate outputs, but they don’t want the data to be shaping future versions of the model. Is data anonymised when training the model? Can data be deleted? Are there humans reviewing outputs? How long is customer data being retained by the application vendor? Are there any other security guardrails and are outputs consistent or will they be unpredictable?
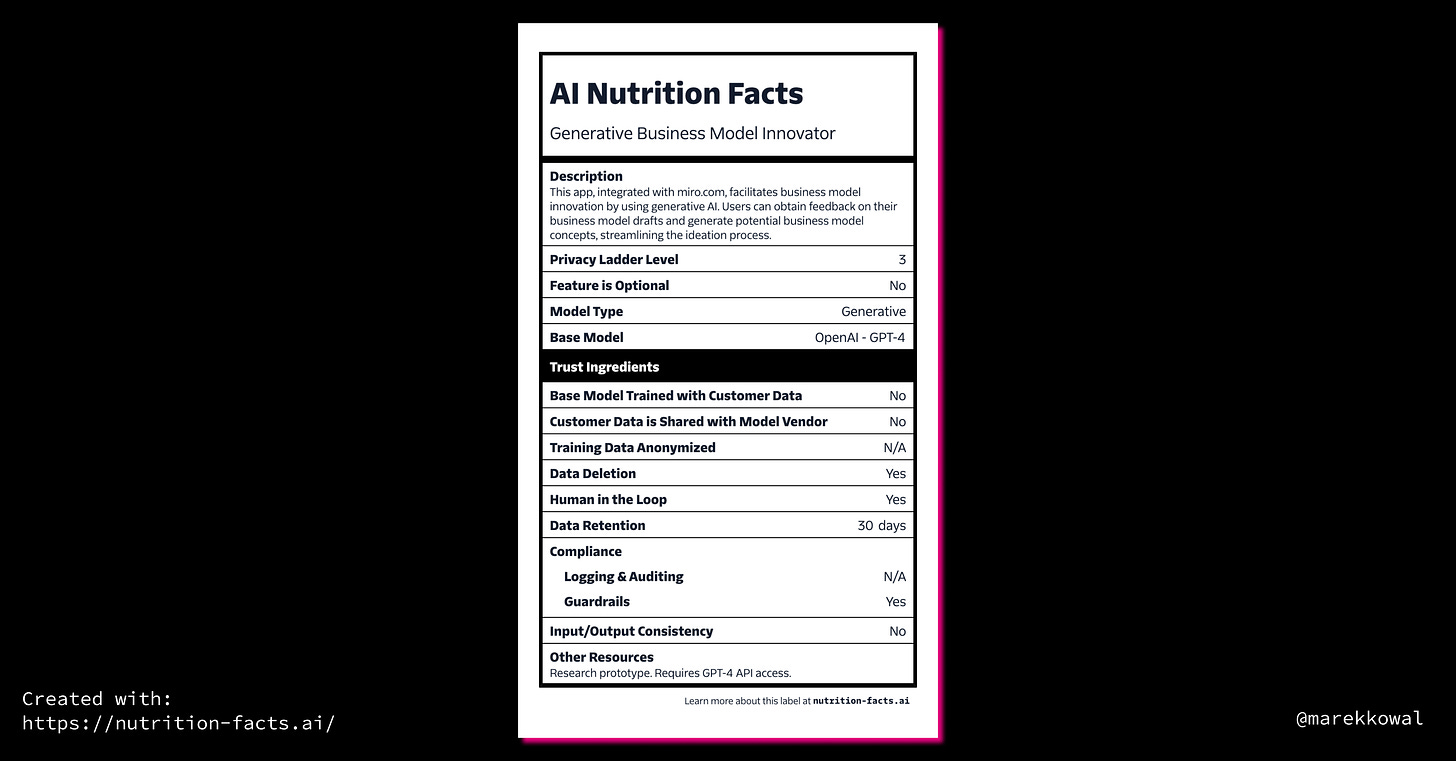
This transparency is a potential game-changer and much needed in our current landscape. Lack of transparency was a significant issue for our team when we were unable to use OpenAI’s API. A clarification on how customer data was used was finally provided in a CNBC interview with Sam Altman. But is a media interview statement good enough for us to proceed with using the sensitive data? If OpenAI had a label like this one for its API, we wouldn’t have had to guess what they were doing with our data!
What remains unclear is the pathway to consistent use of such labels. Should they be legally enforced, in the same way nutrition information or energy efficiency labels are, or shall we rely on voluntary self-labeling by the industry? Is self-labeling the approach, or should labels be created by independent entities? Should the labels be used for applications, or for features within applications?
There is no doubt we need clarity and transparency about Generative AI. Such labels can help us, the end users, decide how and when to use Generative AI responsibly. Or, at least, remind us what to pay attention to when we use these tools.