Poetical Science
Earlier this month, I spoke at Queensland Artificial Intelligence Hub about the history of Generative AI and called for more transparency when sharing algorithmic outputs. I received great feedback after the talk, and I thought you might like it too. While there isn't a video available, I made an audio recording that you can tune into below. Or, you can read the write-up of my speech (not just a transcript) below.
If you decide to listen in, you might still find some of the images below helpful. Enjoy!
1. The Roots of Generative AI
It’s fascinating how history intertwines the brilliance of past visionaries with today's groundbreaking innovations. Our journey today starts not in the 21st century but way back in the 19th century.
While most might credit Silicon Valley or recent tech hubs for the genesis of generative AI, the tale stretches back to Charles Babbage. In 1839, Babbage conceived the idea of the first mechanical, general-purpose computer, the Analytical Engine, which, though never realized in his time, paved the way for computer pioneers.
One such pioneer, Babbage’s contemporary, was Ada Lovelace. Ada was the daughter of the poet Lord Byron and Lady Byron, a highly educated woman called by her husband “the princess of parallelograms”. Ada combined poetic flair with analytical insight. On learning about the Analytical Engine, she wrote: “The Analytical Engine weaves algebraic patterns, just as the Jacquard loom weaves flowers and leaves.”. And she coined a term to describe the beauty of algorithmic processing: "Poetical Science."
Through her writings, she postulated that the Analytical Engine could go beyond mere calculations. She wrote words that seem eerily predictive of today’s Generative AI: “The engine might compose elaborate and scientific pieces of music of any degree of complexity or extent“. This early vision of "computational creativity" resonates remarkably with today's generative technologies.
I often wish we used Lovelace’s term “Poetical Science” instead of the bland “Generative AI”.
2. The Modern Power and Potential of Generative AI
Flash forward to today, and we're living Ada's vision. Generative AI, with its profound applications from crafting intricate designs, giving video production powers to amateurs, curating music, or even shaping unique business models, is redefining creativity. It has democratized innovation, making it available at our fingertips.
But, as we saw with the introduction of GPT-3 in 2020, powerful technology needs to be coupled with intuitive user interfaces. It wasn’t until OpenAI turned generative AI use into an experience as seamless as Google search through ChatGPT that its widespread adoption began. Other Generative AI systems, such as Midjourney, are just as easy to use—as easy as interacting with humans on a social media channel.
3. The Double-Edged Sword: Accessibility vs. Misuse
However, universal accessibility is a double-edged sword. On one side, we have the democratization of technology, and on the other, the potential for misuse or misunderstanding.
Take, for instance, the "robodebt scheme" incident in Australia. For those unfamiliar, this was a contentious government debt recovery program. Using algorithms, it aimed to match income data and discern discrepancies. Instead, due to flawed implementation, following a flawed approach, it falsely accused hundreds of thousands of Australians of incurring debts. A stark reminder of the unintended consequences of unchecked AI, even though “robodebt” was not really AI. The more we learn about robodebt, the more we understand the destructive role of humans in the process, but at the same time, the amplifying role of algorithms in the scheme.
While robodebt is not an example of the impact of Generative AI, we’re already seeing Generative AI amplifying our sloppiness!
4. A New Era of Transparency and Trust
In my own experience, even school reports aren't exempt from the encroachment of algorithmic generation. While it's heartening to see educators use technology to save time, knowing when and how it's applied becomes crucial. Transparency isn't just about trust; it's about understanding the boundary between machine efficiency and human touch. I have nothing against teachers using Generative AI to speed up report writing, but I would love to know when they do it.
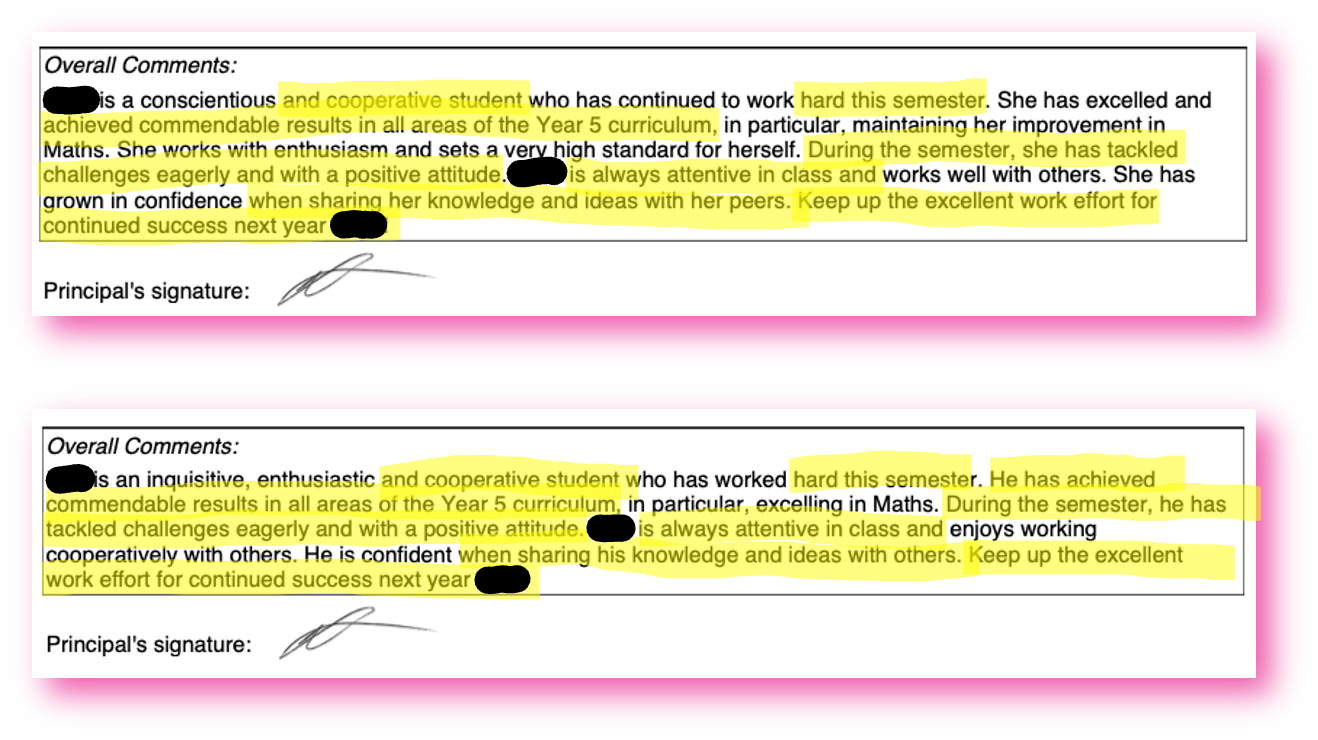
Could we, inspired by the tiered certifications of autonomous vehicles, advocate for similar transparency in generative AI's outputs? Envision a world where AI-generated content comes with clear labels, signifying the depth of human versus machine involvement. These automation levels below are inspired directly by car automation levels, but applied to algorithmic work.
5. A Plea for the Future
In our rapidly evolving digital landscape, marked by the prowess of generative AI, there's an urgent need to annotate algorithmic outputs in some areas of our lives. Every piece of content that has an impact on our life—an administrative decision or a school progress report—should come with clear indications of its origins: is it purely machine-generated, human-crafted, or a combination of both?
Such annotations would not only enhance trust but also offer context. When we consume content, we have a right to know the 'who' or 'what' behind its creation. Clear, identifiable markers can help us understand, appreciate, and critique content more accurately. As we embrace the world of generative AI, let's ensure we also champion a culture of clarity and transparency.