We couldn’t predict everything that would happen. And we didn’t. There are a few things we got quite wrong indeed. The challenge of predicting the future is that there are so many factors that can influence how it unfolds.
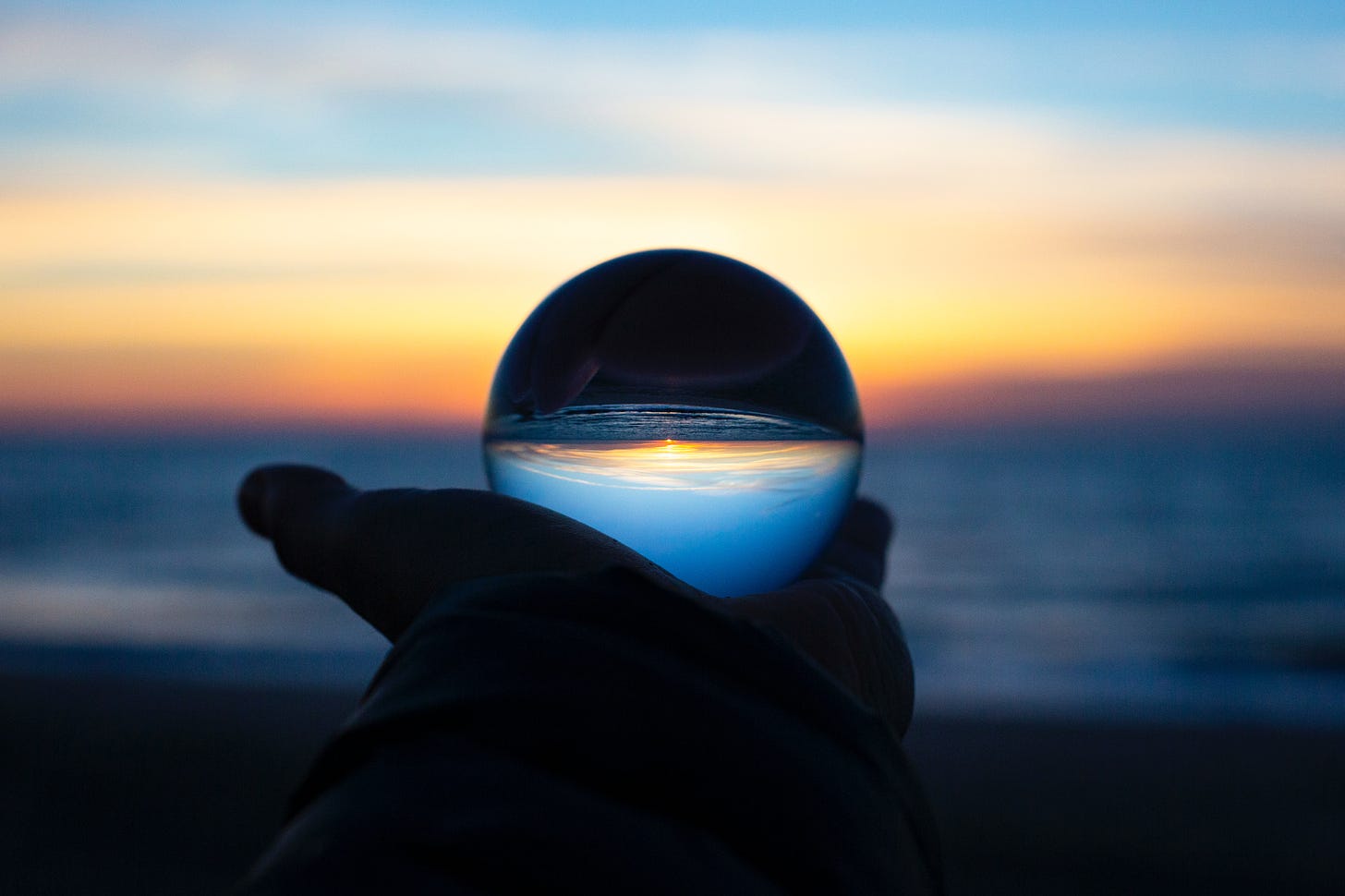
The end of sharing economy: From what’s mine is yours to what’s yours is mine.
The things you own end up owning you. Rachel Botsman, 2010
One of the more exciting futures we were expecting was one where our assets would be used in many new ways, generating value for us. In 2010, Rachel Botsman wrote “What’s mine is yours”. In her book, she was directing our attention to a powerful social trend, powered by the available technology: the sharing economy. Unfortunately, what started as “what’s mine is yours”, has gradually shifted to “what’s yours is mine”.
Back in 2010, seeing young and exciting startups like Airbnb and Uber, we were expecting the “economy of people” to rise. Sharing economy was meant to bring communities together. The technology platforms were meant to allow individuals to interact more easily and curb high consumption of products used only occasionally. Instead, the most typical value proposition of sharing economy platforms these days revolves around price, efficiency, and convenience. The community became a commodity, as April Rinne wrote. Instead of the sharing economy nirvana, we have been witnessing a rise of data and resource-hogging monopolies who realised that they could grow by getting individuals to give them all their assets. TechTarget’s Tom Goodwin said: “Uber, the world’s largest taxi company, owns no vehicles. Facebook, the world’s most popular media owner, creates no content. Alibaba, the most valuable retailer, has no inventory. And Airbnb, the world’s largest accommodation provider, owns no real estate. Something interesting is happening.” Perhaps except Alibaba, who in this context is an extremely efficient orchestrator, all the other organisations are simply making a profit on what they don’t own (vehicles, our stories, our real estate).
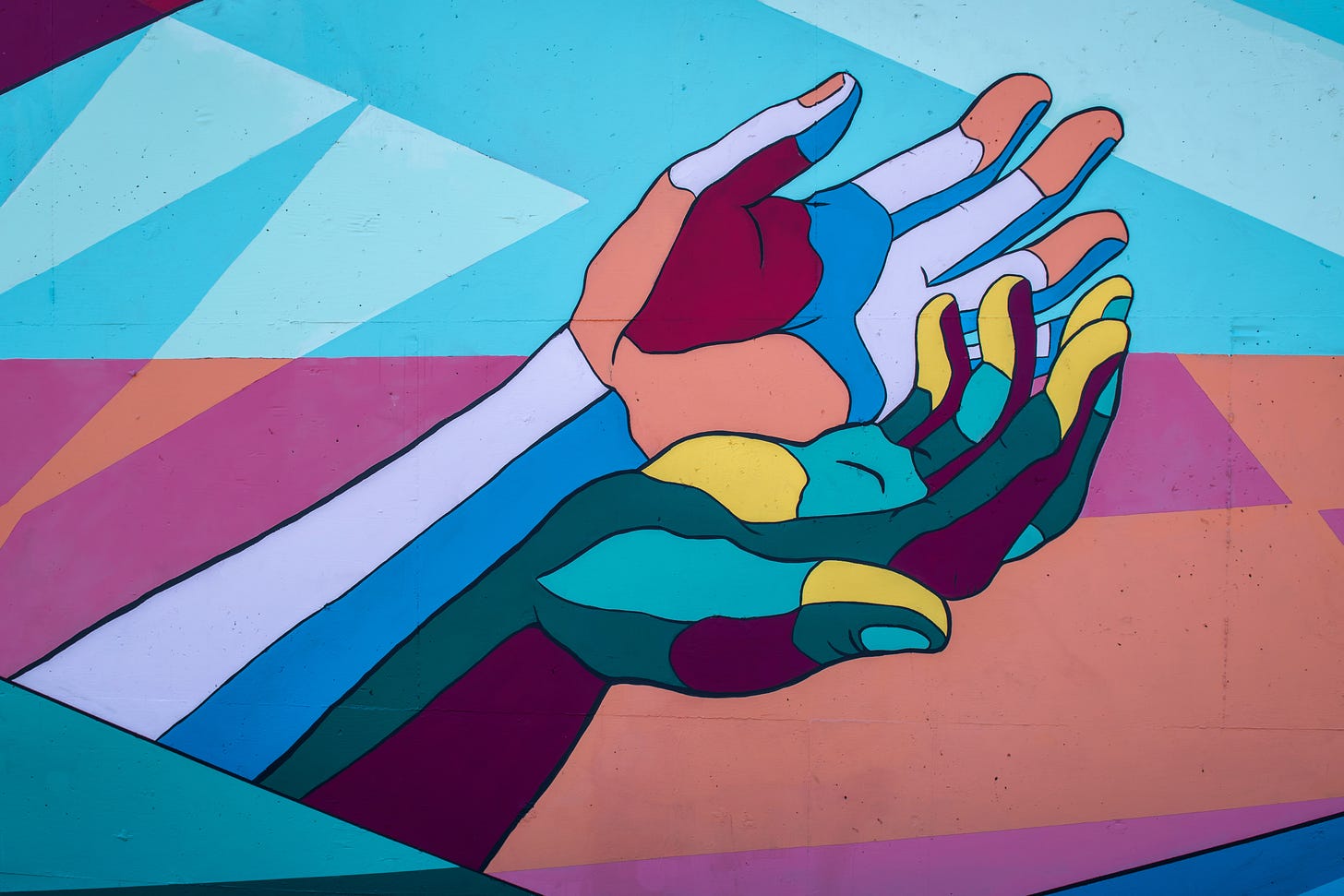
We need to look hard outside of monopolies and profit-generating machines to find examples that are true to the original intentions. One such example is Kiva.org: a platform for microloans for global underserved communities.
The end of trust in algorithms: Weapons of maths destruction.
Computers are like a bicycle for our minds. Steve Jobs,
The tech pioneers started with a noble goal: to build technologies amplifying human ability. Computers, controlled by algorithms, have massively increased our outputs. Digital machines are very good at following our directions. They never get tired and rarely make mistakes. If they do make mistakes, it’s almost certain that it’s a result of our mistake—either something’s not right with the algorithm, or the data is wrong. As long as we were building so-called deterministic systems - technologies that follow our intentions expressed through step-by-step instructions, everything was mostly fine, though. Any mistake in an algorithm’s behaviour, once spotted, could be easily fixed. In recent years, however, we realised that for particular types of problems, this approach didn’t work anymore. It’s impractical, if not impossible, to specify step-by-step how a computer vision algorithm should look for cancerous cells in a microscope image. But an application of the so-called convolutional neural network leads to accurate detection. Such algorithms often referred to as machine learning algorithms are not explicitly “configured”. Instead, they rely on large amounts of data to configure themselves. The cancer-detecting algorithm first had to learn from 8’000 images, annotated by humans, to get to a point where humans are not needed to detect cancerous cells anymore. Now, imagine training a loan approval algorithm on hundreds of thousands of loan decisions from the past. If these decisions were biased (for instance bank officials preferring granting loans to men than women), or—in other words—the training data contains unintended behaviours, these are then engrained in the machine learning algorithms. Simply speaking, if training data is sexist, the machine learning algorithm becomes sexist too. And unless we are aware that this might be an issue, or are trained to look for such cases of bias, we might not be able to find it out until it is too late, which is exactly what Apple experienced in late 2019.
When Steve Jobs referred to computers as bicycles for our minds, he was suggesting that they help make us more efficient. Better use our energy: whether it’s our creative energy, computing skills, or perhaps other forms of expression. However, a bicycle does not decide for us where to go. In the past years, we have been giving algorithms increased autonomy in complex situations, and in many cases, they now take the lead. Assessing the risk of reoffending in court proceedings, loan decisions, or—in some countries—welfare payments, are only some of the examples where algorithms have a lot of autonomy and wreak a lot of havoc, these days.
Thankfully, we are now starting to realise this is an issue. We hear calls for banning black box algorithms in certain applications, see researchers auditing them. There are calls for black-box algorithms to be clearly labelled as such, or used only with a human validating their results. Europe introduced the right to explanation, in its General Data Protection Regulation, ensuring that any subjects of algorithmic decisions can receive sufficient explanation of any decisions they’re a subject of.
Will these changes help us regain trust in algorithms? We will see.
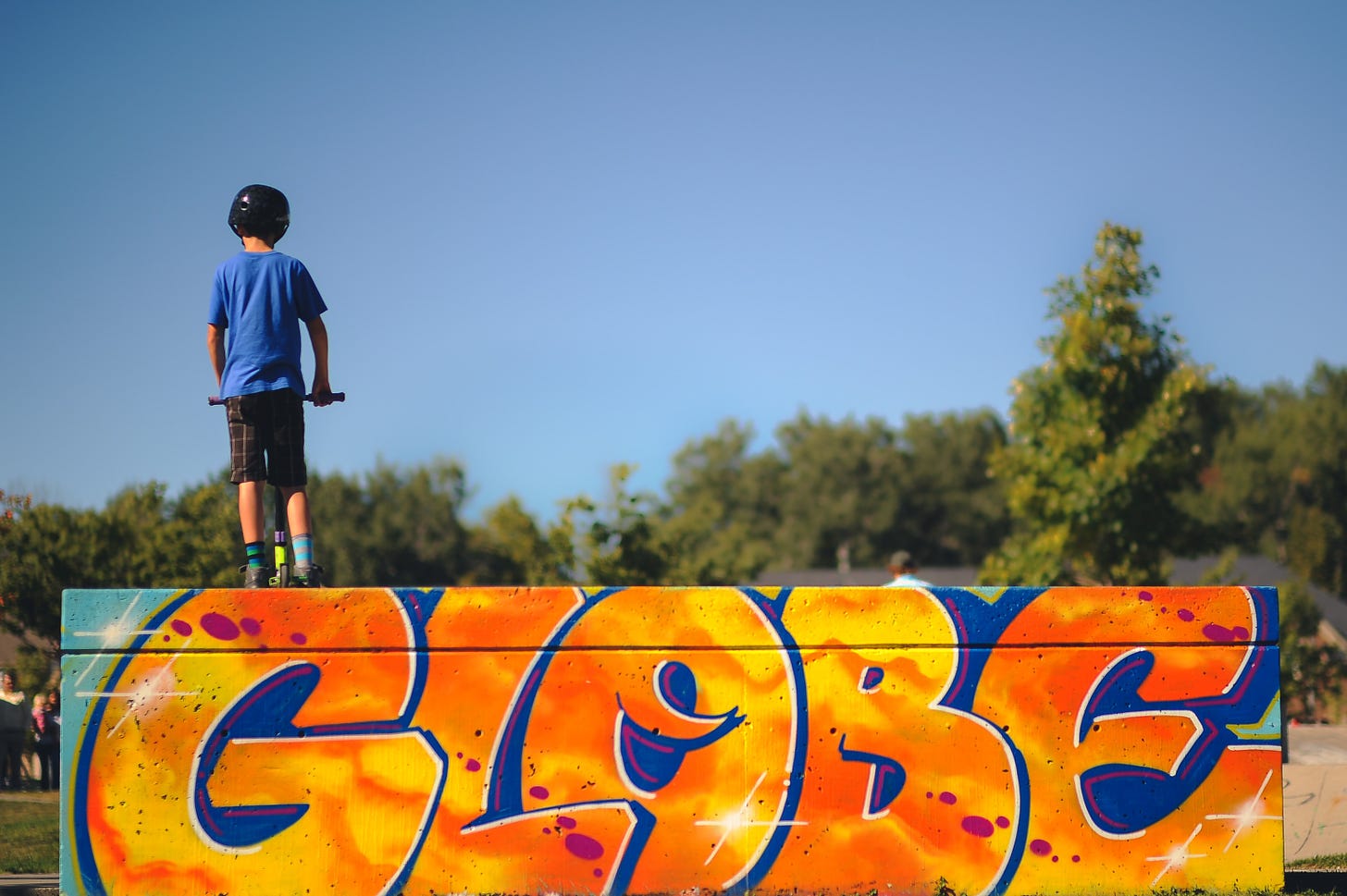