10 ChatGPT governance checks
How to ensure the proper use of ChatGPT—and similar tools—in business?
Missed opportunity
In August 2020, in this newsletter, I wrote: “Imagine living in a world where it is impossible to tell a human output from the creation of an algorithm. Some of us will love this new world. Some of us will be very concerned about it.”
This was my take on GPT-3, the technology (called a large language model) behind ChatGPT.
I also wrote: “Think about poor teachers trying to tell whether an essay was written with the help of an algorithm“. Now, two and a half years later, we are witnessing just that. Unfortunately, despite plenty of signals in the past, the education sector has been caught by surprise. In Australia, only one state, South Australia, is embracing the use of artificial intelligence in classrooms, while the other states decided to block access to ChatGPT for students1.
Any such blanket blocks are futile. There are several alternative tools available to students right now. There’s YouChat, which many believe is better than ChatGPT because it can access the Internet to enrich its responses. It’s also super easy to use. Yesterday, Google announced Bard—its own response to the competitive threat of ChatGPT. And it looks like tomorrow, Microsoft will make a big, ChatGPT-related, announcement too—the heat is on.
So, in a home-schooling style, I am now teaching my kids how to work with GPT-3, DALL-E, and Midjourney. Just like they had to learn to use a calculator, they now need to learn to use generative AI tools. Such a shame they can’t use these revolutionary technologies at school. Yet2.
The governance of large language models
ChatGPT is already transforming industries and will continue to do so. I don’t need to give you many examples here: your social media feed is likely full of those. Last year I wrote how I delivered a conference presentation—to school principals—that was written entirely by GPT-3 (I told every school principal and teacher in the room to prepare for GPT-3 entering their classrooms)3. I also shared how my team used GPT-3 to turn our research into stories, saving us days of work. I am sure your business is teeming with employees using such tools to speed up their work, even if you don’t know it yet (in my upcoming book—stay tuned—I call it shadow automation).
Over the last three years, I have learned a lot about working with large language models. The biggest lesson? They are best at being impressive. But once you move past the “wow, this is unbelievable” stage, you will start noticing all sorts of issues with the outputs. The content might be untrue, biased, illogical or even offensive. The delivery might be unreliable. Or the tool itself might be banned in a particular situation.
I have developed a list of “checks” to deal with these issues. Think of these as “ChatGPT governance checks”. They will also apply to other large language models.
Whether you love this new world or are very concerned about it, sooner or later, you will be exposed to a tool like ChatGPT. And when you are, it’s a good idea to keep these checks in mind4.
Checks 1-4: Should I use ChatGPT?
Before using ChatGPT for a task at work, you need to establish whether you can and should do it. Is it allowed to use ChatGPT (permissibility)? Is it the right tool for the job (suitability)? Are there any concerns about how your data would be processed and stored (privacy)? Do you have processes in place to avoid undesirable outputs (accountability)?
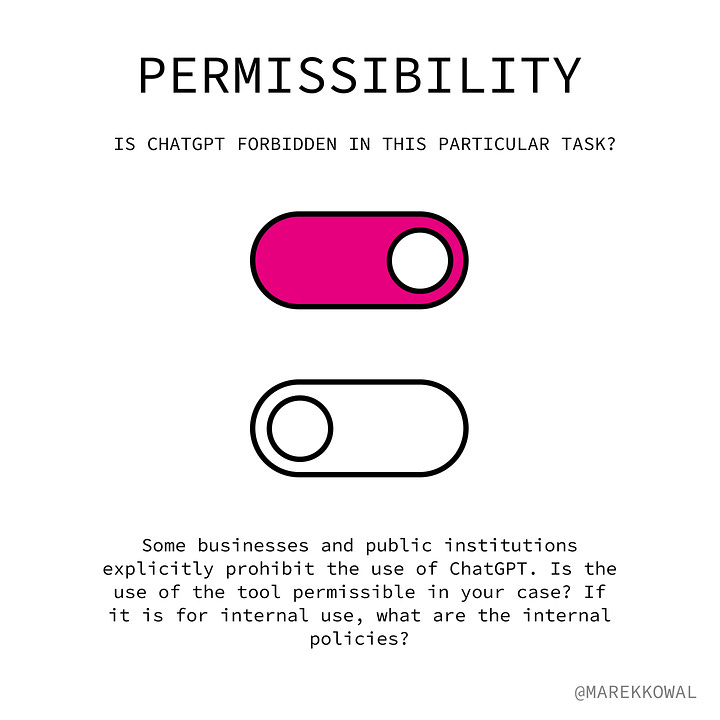
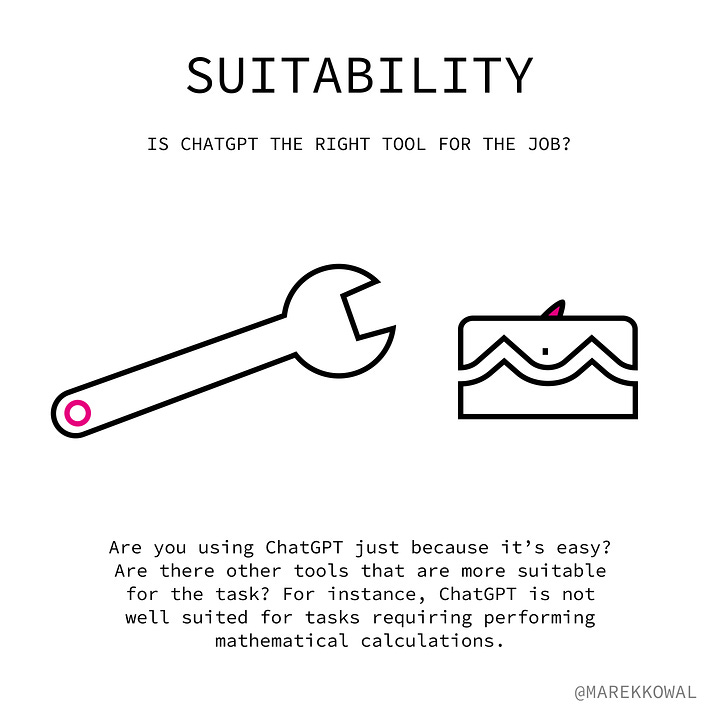
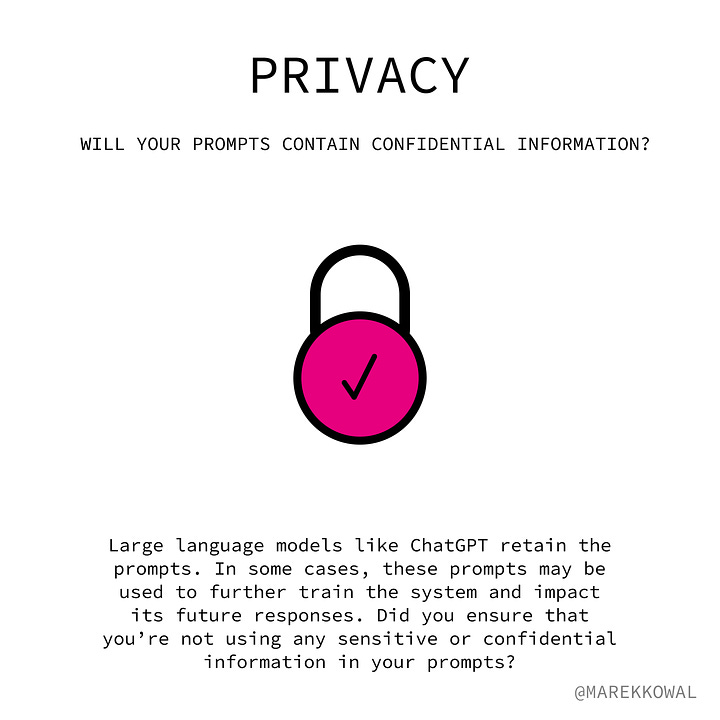
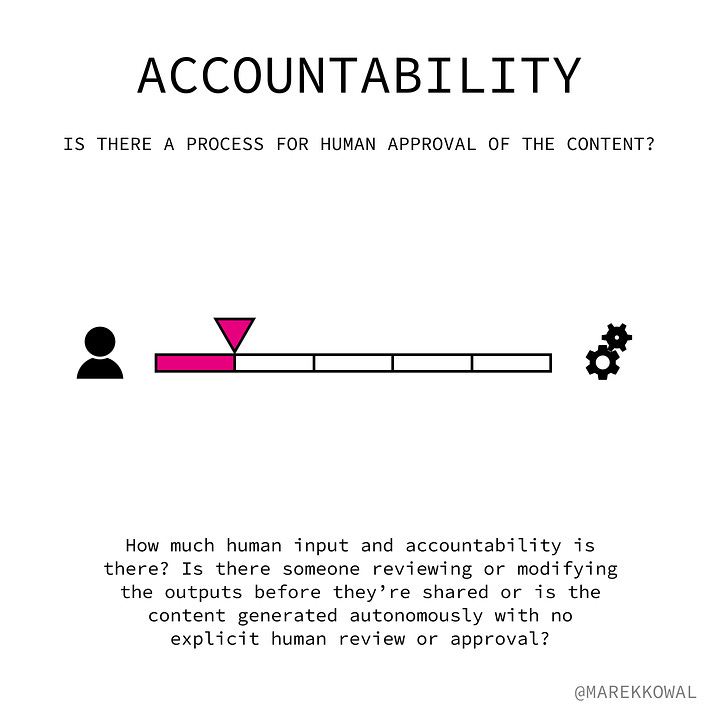
Checks 5-8: What to keep in mind when using ChatGPT?
Once you establish that you can and should use ChatGPT for the task, time to think about the output itself.
Large language models are pretty "creative" in what they write. Some are better at ensuring what they write is not entirely made up, but it’s important to always check for factual correctness (truthfulness).
There have been a lot of examples of bias encoded in ChatGPT and similar tools. The models are continually improved, but it's better to assume there will always be some bias. Try to identify and remove or edit any questionable outputs (lack of bias)
Large language models create very impressively looking outputs. But once you scrape off the shiny coating, lack-of-logic issues often emerge (common sense). In my experience, addressing this space is the most time-consuming.
Additionally, consider disclosing the use of a large language model (transparency) unless there is a justified reason not to do so. For the record, I didn’t use a large language model to write this post.
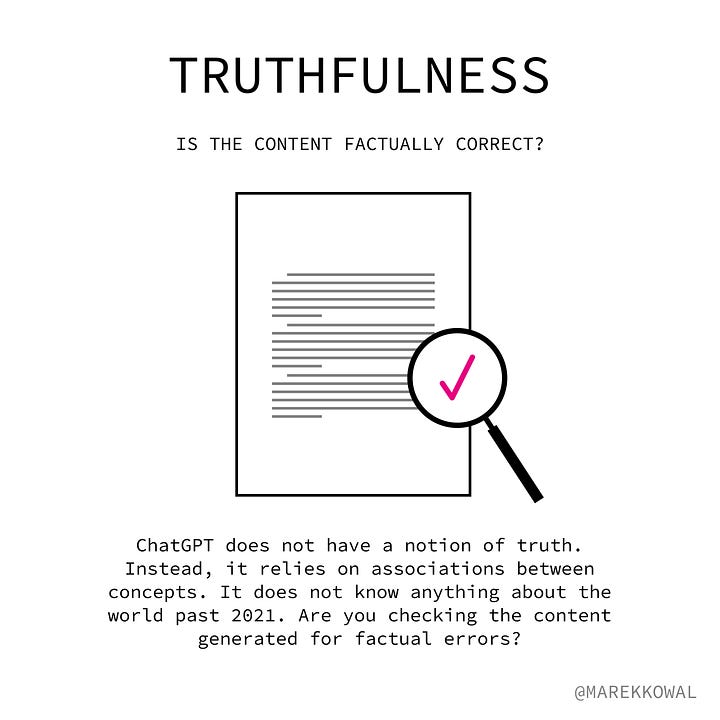
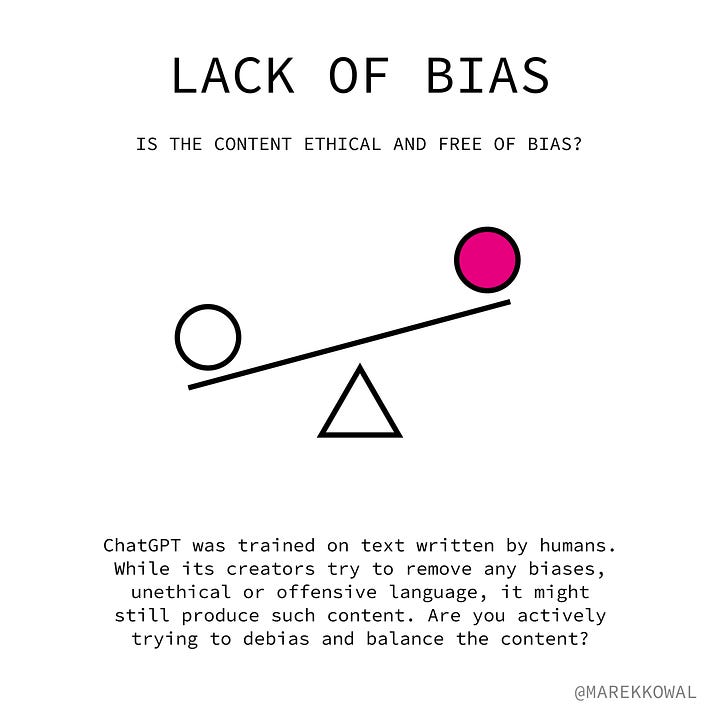
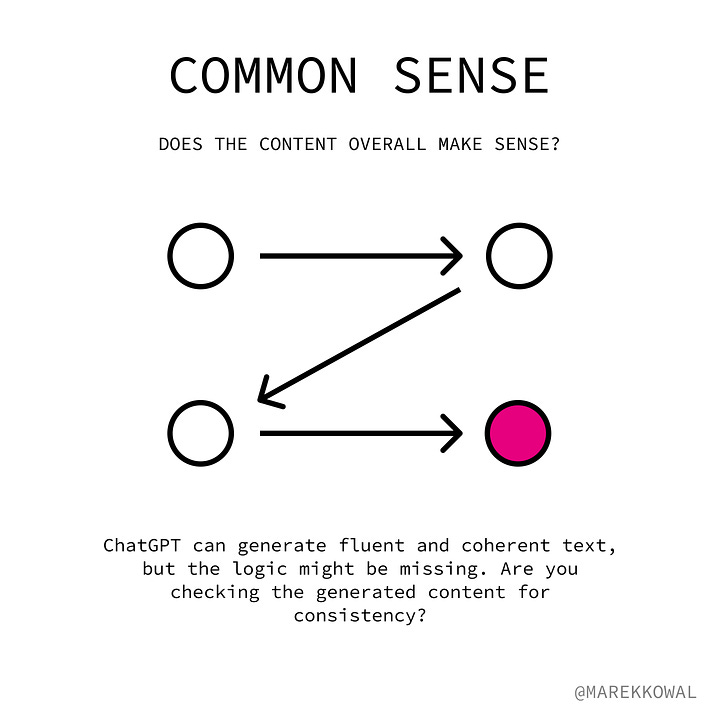
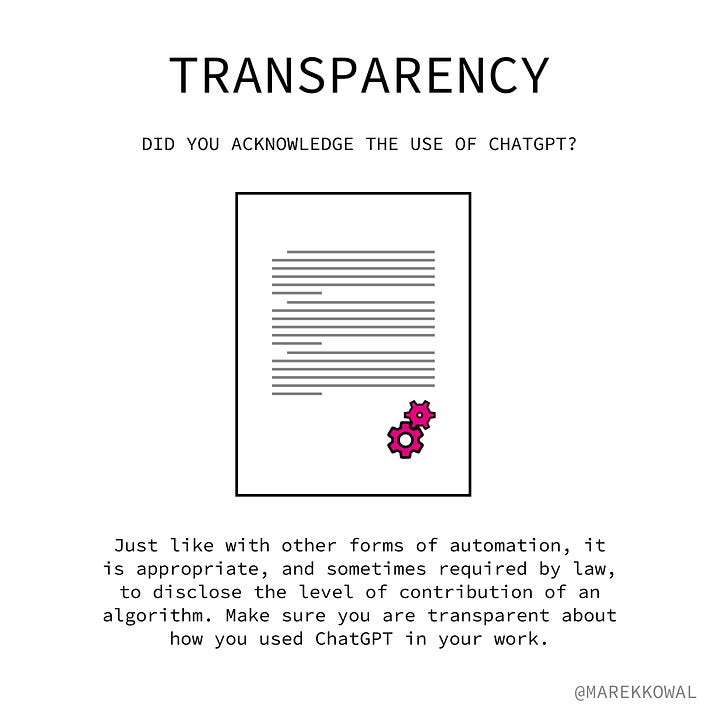
Check 9-10: Ongoing considerations.
When you integrate an algorithm like ChatGPT into a process, there is a risk that your employees (or you) become too reliant on it. You need to plan for a situation where the tool is not available. Is there a risk that you will lose relevant skills over time and suddenly be unable to continue your work if the model fails? Good to have a backup plan (self-reliance).
Finally, one thing I learned about working with LLMs is that they can always surprise you. They will throw unexpected content at you when you suspect it the least. Remaining ready to step in is critical, and it never gets boring.
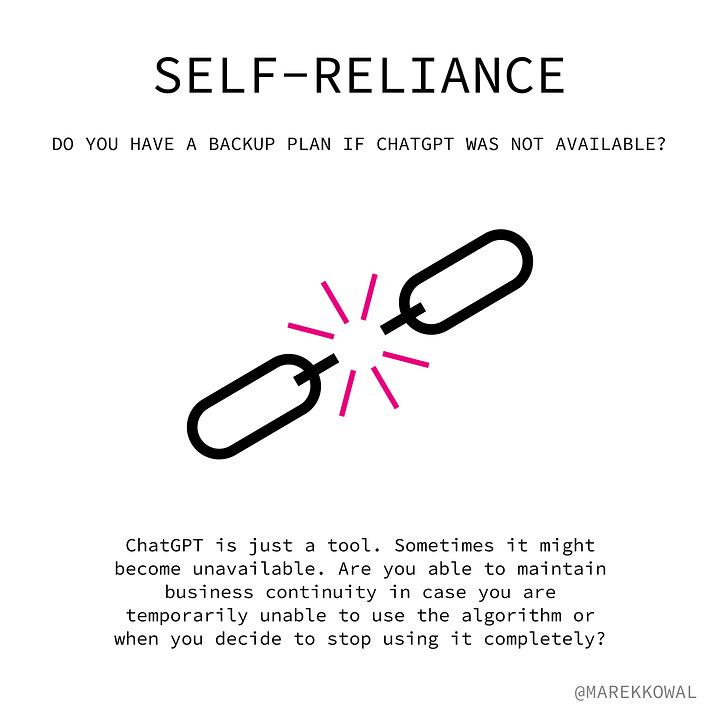
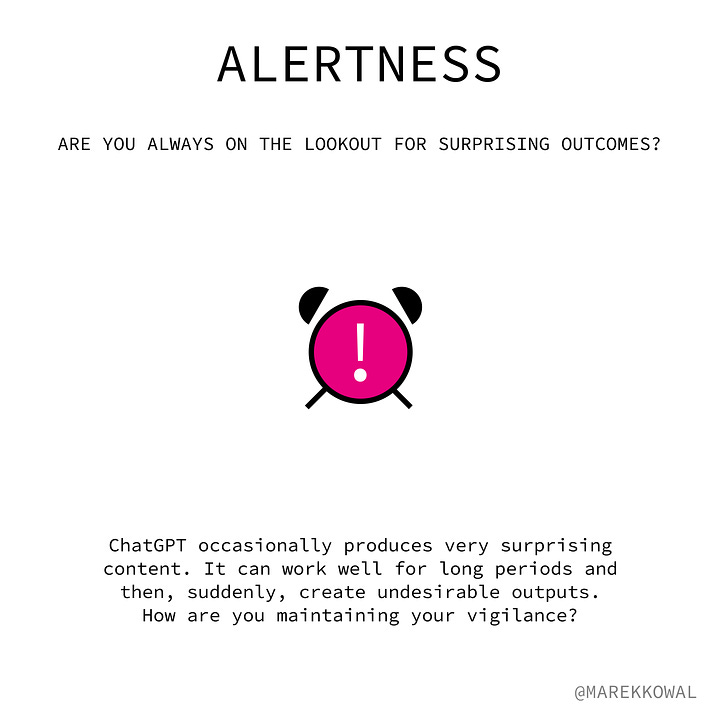
You can download all these rules as a PDF below.
I hear that the main reason that ChatGPT is currently blocked is because of its requirement for the users to be 18+ and that in some jurisdictions, there’s a requirement for any data to be stored on-shore (see the “privacy” check). I have a bottle of vodka in my freezer, and I found that telling my kids not to drink it is enough. I don’t need to put a lock on the freezer. I also have another way of telling whether they tried the vodka, but I won’t disclose it here. Who knows, my kids might be reading the newsletter (hi, did you brush your teeth?).
This will change when they enter a university or the workforce. For the next edition of an executive MBA unit I teach, I expect my students to use generative AI tools in their assignments. Not using AI will put a student at a disadvantage.
Pro tip: don’t deliver GPT-3 written presentations live. Just don’t. My brain was hurting for the next few days from trying to bring the story coherently together while on stage.
I shared an initial version of these checks in a LinkedIn post, which helped me refine them. Theresa Lauf suggested adding a privacy check, and Kristine Dery convinced me that “checks” is a better name than “rules” (which I used initially).